Three challenges for AD – and how CARIAD is solving them with software
Safety and reliability are the most important requirements for automated driving. In this article, we explain how the use of three parallel systems can help in this area and revolutionize future automotive mobility.
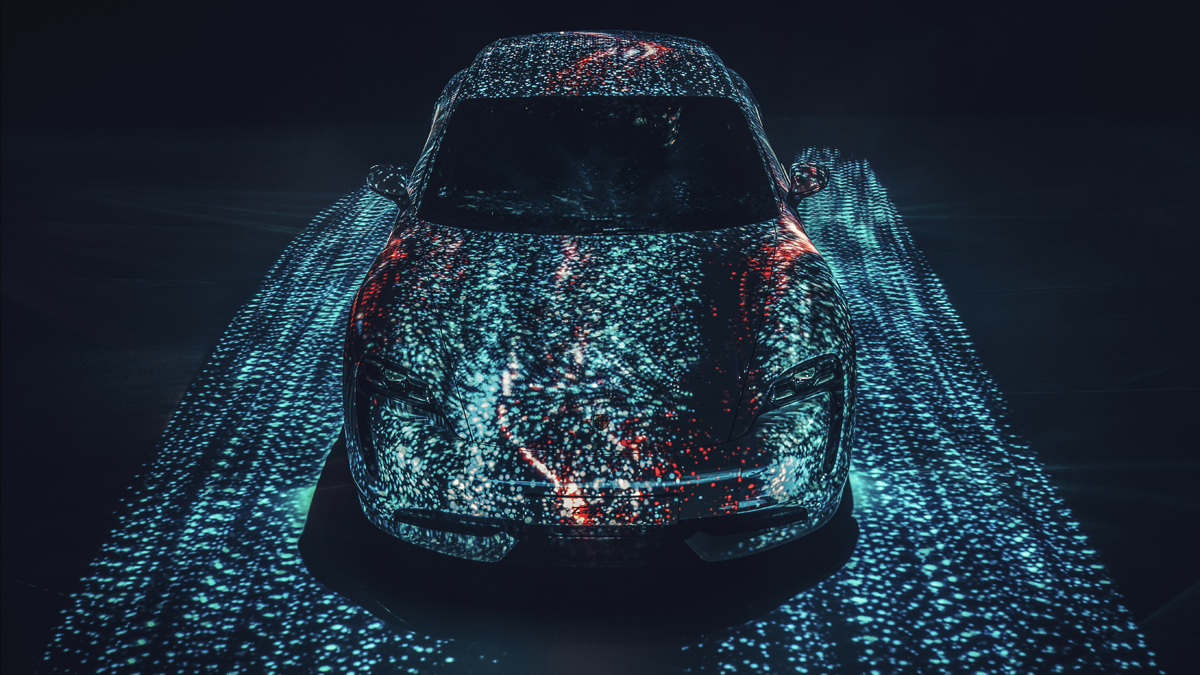
At CARIAD, our test vehicles continuously collect and process data to improve the safety, reliability and robustness of highly automated driving functions. This means that they’re prepared for all situations on the road, no matter how unusual. In an article for the Porsche Newsroom, CARIAD System Architect Andreas Nagler recently explained how specific challenges for automated driving can be overcome. He emphasizes the need for a new mindset, since vehicle software will be constantly evolving in the future – much like smartphones today. The following overview shows solutions to make automated driving as safe as possible.
Challenge #1: Mastering all imaginable driving situations
With redundant, strictly separated systems, the vehicle is able to switch between different trajectories while driving. The goal is to react safely and reliably in all imaginable situations. The overall system developed by CARIAD is therefore able to function regardless of vehicle type or driving style. What’s more, in reality, conditions are not always ideal. Poor roads, weathered lane markings and abrupt changes in the behavior of other road users can pose a challenge to automated driving. For example, if cargo breaks loose from a truck ahead, a quick decision must be made whether to brake or swerve. What would cause a moment of shock for human drivers is handled by three parallel systems in the highly automated vehicle.
The ‘main planner’ takes care of the regular driving operation in a comfort-oriented manner, braking and accelerating smoothly. At the same time, the ’fallback planner’ calculates a trajectory that brings the vehicle into a safe position if necessary. These two systems are constantly checked by the ‘supervisor’ that always selects the safest alternative. In the unlikely event that the main planner misses an obstacle, the fallback planner responds safely by braking or swerving.
So, instead of having only one system steer the vehicle, so-called ‘decomposition’ is used. Interruptions are prevented in this way. For the systems to work smoothly, they need a database – in a similar way to how a human driver attends a driving school and gains experience in dealing with dangerous situations such as aquaplaning over the course of their life. But humans don’t always react quickly enough – automated driving, on the other hand, does.
Challenge #2: Minimize ‘common cause’ errors and create true redundancy
These three systems – the main planner, fallback planner and supervisor – must be technically separated from one another, each with its own hardware, software and data sources. Otherwise, ‘common cause’ errors can occur because a change is misinterpreted by all three systems.
"So, to achieve true redundancy, it's important not just to copy systems," says Andreas Nagler. For example, the supervisor works with an object list to get a picture of the environment. To do this, the radar sensor scans all detectable nearby vehicles and objects, including the direction of movement. While the supervisor operates with the object list generated by the sensors, the two planners work directly with the raw data, such as point clouds from laser scanners (LiDAR).
The main and fallback planners also take advantage of what’s known as sensor data fusion. If only one sensor reports an object and all other sensors do not, the algorithm may decide that this signal can be ignored. The supervisor, however, considers the sensors in a strictly separated manner. Therefore, the individual systems each form their own impression, which, when combined, ensures safer behavior.
Challenge #3: Calculating the traffic flow
Weighing up potentially dangerous situations requires highly complex software that has to decide within seconds whether to react and, for example, change lanes unnecessarily and over-cautiously. Here, the software acts both directly and indirectly. The supervisor checks the paths calculated by the planners and predicts the path of travel for the next few meters, as well as a few seconds into the future. The second prediction is much more complex. It depends, among other things, on speed, road construction, weather conditions, and of the way in which other road users have driven in the past. The supervisor compares this with the trajectories of the path planners and changes them if the so-called ‘sovereignty zone’ around the vehicle should be violated.
Further challenges: Automated parking
Not only is there plenty of room for innovation on roads; parking is also an essential element in automated driving. For example: Will parking garages themselves take remote control over cars? You can find out more about upcoming possibilities and challenges – like preventing unauthorized access to a vehicle – in this Porsche Newsroom article.