Chats with Your Car: Integrating Large Language Models on Wheels
Large language models are making cars smarter. In our discussion with Peter Schlicht, Head of Artificial Intelligence at CARIAD, we explore the factors that come into play when implementing these models, considering the advances in AI that are reshaping the interactions with our vehicles.
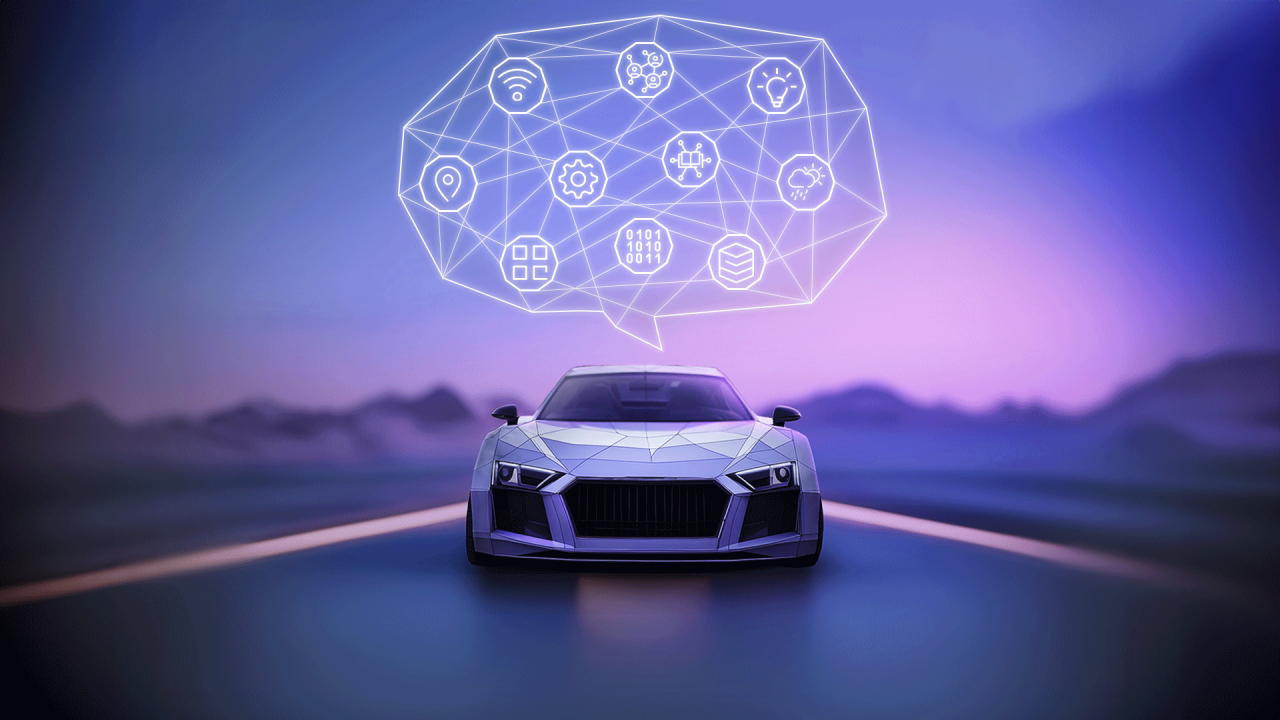
You are cruising down the highway, when you casually ask: “What do you think the weather is going to be like in the afternoon?” The response is natural – except it’s coming from your car.
This is the world of Large Language Models (LLMs) offering intuitive virtual assistance—a game-changer for in-car interactions. In his role, Peter is particularly interested in ensuring safety and efficiency both in development and application of automotive AI – Generative AI and foundational models such as LLMs make this field even more exciting.
What is a Large Language Model?
In simple terms, Large Language Models (LLMs) are Artificial Intelligence Systems trained on vast amounts of data, hence the term “large.” Built on machine learning techniques, they can recognize questions, generate answers, and perform a variety of other tasks just like a human.
Is it important for companies build custom technology with LLMs for more accurate interactions?
“Creating a custom language model from scratch is resource-intensive and complex. However, organizations have other efficient options: leveraging cloud-based interfaces, such as ChatGPT, for example” says Peter.
By adapting existing models to the organization’s specific requirements and fine-tuning them with additional data, companies can strike a balance between cost-effectiveness and customization. This approach, known as Retrieval Augmented Generation (RAG), combines the strengths of pre-trained models with domain-specific knowledge.
Moreover, organizations can also tap into pre-existing open-source language models, building upon established foundations instead of starting from scratch. These models can be customized for specific tasks or domains, ultimately saving time and resources.
What factors should be considered when using LLMs in customer applications?
The integration of ChatGPT, an OpenAI language model, into the Audi Q6 e-tron’s infotainment system is one example of a large language model building the foundation for a customer application. When working with LLMs, managing their responses becomes crucial. Since we cannot predict what these models might generate, a combination of pre-processing and post-processing techniques are used to enhance the quality and relevance of their outputs. System prompts play an important role in shaping LLM behavior. Sticking with the weather example, this could be: Tell the driver about the weather forecast this afternoon. These initial instructions guide the AI model’s understanding and context and help ensure that responses meet organizational quality standards. LLM responses are constantly refined, including fine-tuning of system prompts and post-processing based on feedback. It is an iterative process.
Can all output generated by LLMs be trusted?
While LLMs can produce impressive output, a recognized challenge is for them to ‘hallucinate’ answers. The quality of LLM results heavily relies on input data quality. To identify and fix inaccuracies, a systematic framework is essential. It is important to curate data and establish appropriate guardrails. New techniques across the market today can identify confabulations—arbitrary and incorrect outputs—by assessing semantic uncertainty rather than individual word sequences. These methods help customers recognize when caution is required with LLM responses.
What are the methods for running Large Language Models?
The integration of large language models (LLMs) into vehicles is paving the way for personalization, but there are operational challenges to consider. Due to their size, LLMs often run in the cloud, which impacts interactions. For example, we can’t inquire about specific car details like the interiors as seamlessly as we can with a local system. A local version running directly in the vehicle would provide a more seamless integration, resulting in quicker responses and naturally enhancing the overall user experience.
Are we getting there?
In the future, customers will be able to ask more detailed questions about their vehicles, like the tire pressure but more than that, AI-powered Large Language Models will evolve from voice assistance to include a multimodal approach. Vehicles are getting smarter as we continue to build trust in AI decision making.